Amber | AI-Assisted Bark Beetle Detection
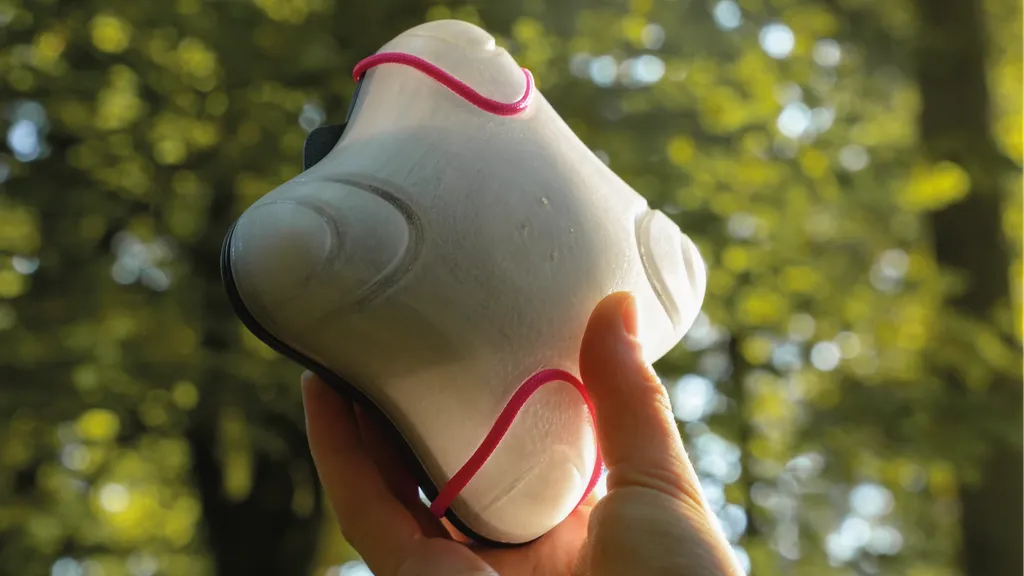
Authors
Bachelor Thesis by Emma Rahe and Levin Budihardjo (in BA Product Design)
Forests play an essential ecological role, but rising temperatures and droughts pose significant risks, including increased bark beetle activity. Amber is an AI-powered monitoring device that records natural sounds year-round and detects bark beetle infestations before they become externally visible. This gives foresters more time to act and prevent the spread. Amber uses seasonal indicators: in summer, it captures beetle feeding sounds in high-risk areas, while in winter, it monitors woodpecker feeding behavior to detect dormant beetles. The AI processes these recordings by converting them into spectrograms, applying image recognition techniques to analyze the visual representation of the audio data. A convolutional neural network scans the spectrogram for patterns characteristic of the target sound. Amber demonstrates how AI can bring significant benefits in areas with seemingly low risk. The AI does not make decisions but highlights natural indicators for foresters. As a flexible, futureproof concept, Amber’s algorithm can be adapted to new contexts, such as biodiversity monitoring or pest detection in fruit plantations.
Summer Semester 2024 Supervised by Prof. Matthias Held and Aeneas Stankowski Supported by FVA Forstliche Versuchs- und Forschungsanstalt Baden-Württemberg